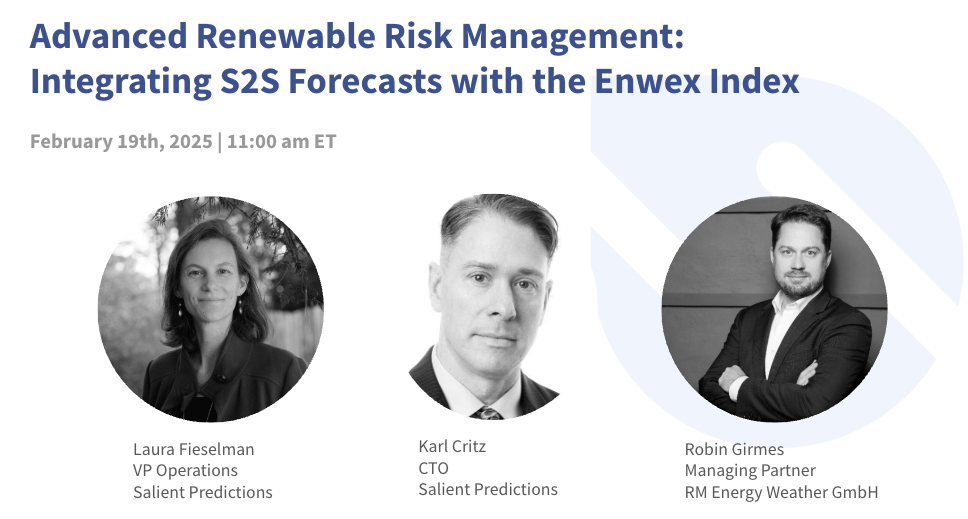
February 14, 2025
February 14, 2025
Diffusion Models for Extending Data-Driven Ensemble Forecasts to Subseasonal Timescales
At the 2025 American Meteorological Society Annual Meeting (AMS), Salient showcased a diffusion modeling approach to subseasonal-to-seasonal (S2S) weather forecasting.

The challenges of S2S forecasting: Why a different approach is required
Forecasting on S2S timescales (2 weeks to 1 year) requires a fundamentally different approach than short- or medium-range weather predictions. Deterministic models become unreliable beyond two weeks due to the chaotic nature of the atmosphere. Meanwhile, traditional probabilistic models — such as numerical weather prediction (NWP) ensembles — often struggle to even beat a simple climatological baseline. In addition, the limited ensemble sizes fail to provide actionable insights, particularly for rare and extreme weather events. For more on this, read Dr. Jason Furtado on the benefits of large ensembles for seasonal forecasting.
When the goal is to compute reliable probabilities for complex weather phenomena, such as simultaneous heatwaves and wind droughts in energy-producing regions, or the likelihood of freezing rain and ice in West Texas, one requires not only a larger number of ensemble members but also physically consistent forecasts.
What are diffusion models?
Diffusion models are a class of generative models that learn to represent complex probability distributions by reversing a noise process. They start with random noise and iteratively refine it to produce structured outputs that match patterns found in observed data.
Why diffusion modeling for weather?
In the context of weather forecasting, diffusion models can generate realistic scenarios of future atmospheric states by learning the relationships between physical variables like temperature, precipitation, and wind. Rather than predicting a single outcome, they model the joint probability distribution of future weather states, capturing how these variables interact over time. This makes diffusion models especially valuable for forecasting rare or extreme events, where traditional methods often struggle to provide reliable probabilities.
However, diffusion models have limitations. Since diffusions models are data-driven, they lack built-in physical constraints and can generate outputs that violate the laws of physics. Additionally, purely data-driven models may struggle with long-range coherence, a crucial requirement for S2S forecasting.
Dig deeper: Diffusion modeling explained at the American Meteorological Society
Watch Fran Bartolić’s recent American Meteorological Society presentation, where he discusses existing weather prediction systems and their limitations. Fran presents a novel modeling framework that combines the diffusion models explained above with a proprietary statistical model to generate skillful ensemble forecasts out to 35 days.
So What? Real-World Applications
For example, in predicting the Texas freeze, preliminary results of the diffusion model accurately capture the probability of extreme events, such as a five-day average minimum temperature below the 1st percentile of historical values. This capability empowers energy traders, utility companies, and agricultural professionals to make data-driven decisions, mitigating risks and optimizing operations.
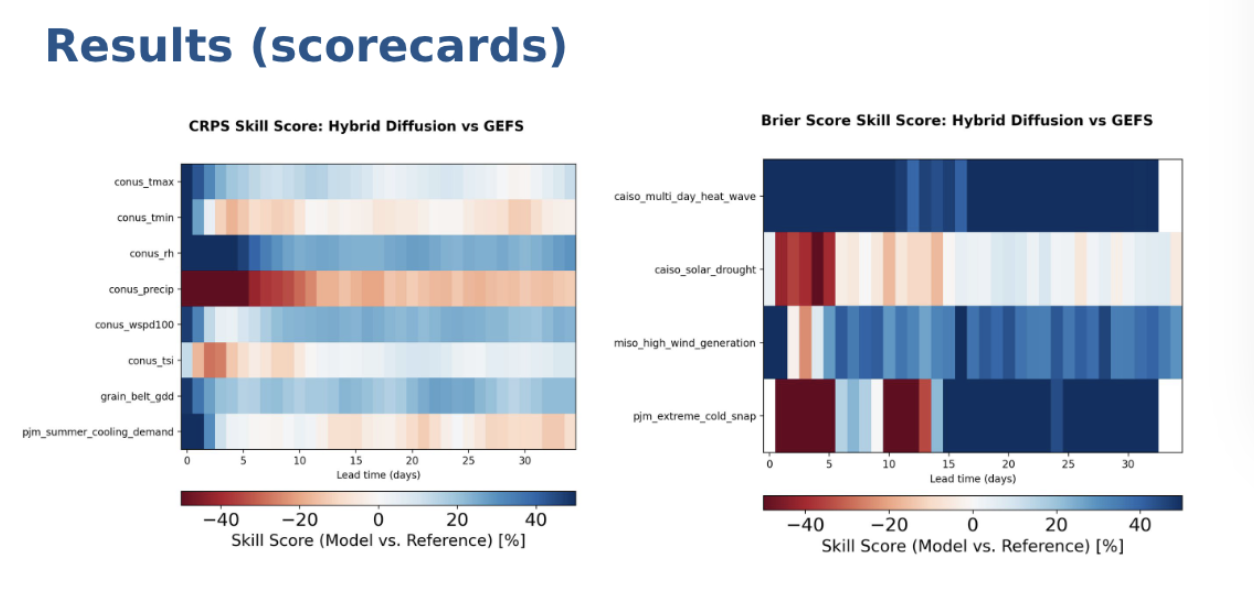
Bonus:
Salient also presented on improving renewable energy decisions support at AMS 2025. Catch that talk by CTO Karl Critz [here].
February 14, 2025
February 14, 2025
Diffusion Models for Extending Data-Driven Ensemble Forecasts to Subseasonal Timescales
At the 2025 American Meteorological Society Annual Meeting (AMS), Salient showcased a diffusion modeling approach to subseasonal-to-seasonal (S2S) weather forecasting.

The challenges of S2S forecasting: Why a different approach is required
Forecasting on S2S timescales (2 weeks to 1 year) requires a fundamentally different approach than short- or medium-range weather predictions. Deterministic models become unreliable beyond two weeks due to the chaotic nature of the atmosphere. Meanwhile, traditional probabilistic models — such as numerical weather prediction (NWP) ensembles — often struggle to even beat a simple climatological baseline. In addition, the limited ensemble sizes fail to provide actionable insights, particularly for rare and extreme weather events. For more on this, read Dr. Jason Furtado on the benefits of large ensembles for seasonal forecasting.
When the goal is to compute reliable probabilities for complex weather phenomena, such as simultaneous heatwaves and wind droughts in energy-producing regions, or the likelihood of freezing rain and ice in West Texas, one requires not only a larger number of ensemble members but also physically consistent forecasts.
What are diffusion models?
Diffusion models are a class of generative models that learn to represent complex probability distributions by reversing a noise process. They start with random noise and iteratively refine it to produce structured outputs that match patterns found in observed data.
Why diffusion modeling for weather?
In the context of weather forecasting, diffusion models can generate realistic scenarios of future atmospheric states by learning the relationships between physical variables like temperature, precipitation, and wind. Rather than predicting a single outcome, they model the joint probability distribution of future weather states, capturing how these variables interact over time. This makes diffusion models especially valuable for forecasting rare or extreme events, where traditional methods often struggle to provide reliable probabilities.
However, diffusion models have limitations. Since diffusions models are data-driven, they lack built-in physical constraints and can generate outputs that violate the laws of physics. Additionally, purely data-driven models may struggle with long-range coherence, a crucial requirement for S2S forecasting.
Dig deeper: Diffusion modeling explained at the American Meteorological Society
Watch Fran Bartolić’s recent American Meteorological Society presentation, where he discusses existing weather prediction systems and their limitations. Fran presents a novel modeling framework that combines the diffusion models explained above with a proprietary statistical model to generate skillful ensemble forecasts out to 35 days.
So What? Real-World Applications
For example, in predicting the Texas freeze, preliminary results of the diffusion model accurately capture the probability of extreme events, such as a five-day average minimum temperature below the 1st percentile of historical values. This capability empowers energy traders, utility companies, and agricultural professionals to make data-driven decisions, mitigating risks and optimizing operations.
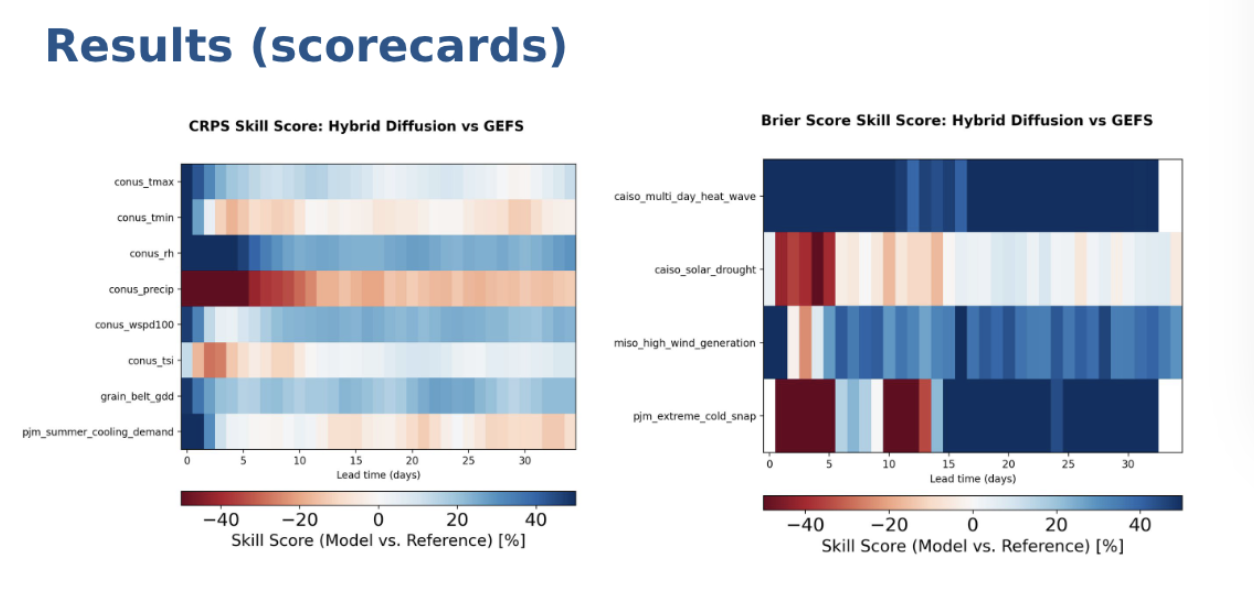
Bonus:
Salient also presented on improving renewable energy decisions support at AMS 2025. Catch that talk by CTO Karl Critz [here].
About Salient
Salient combines ocean and land-surface data with machine learning and climate expertise to deliver accurate and reliable subseasonal-to-seasonal weather forecasts and industry insights—two to 52 weeks in advance. Bringing together leading experts in physical oceanography, climatology and the global water cycle, machine learning, and AI, Salient helps enterprise clients improve resiliency, increase preparedness, and make better decisions in the face of a rapidly changing climate. Learn more at www.salientpredictions.com and follow on LinkedIn and X.