
October 15, 2023
October 15, 2023
Machine Learning Offers New Possibilities in S2S Forecasting
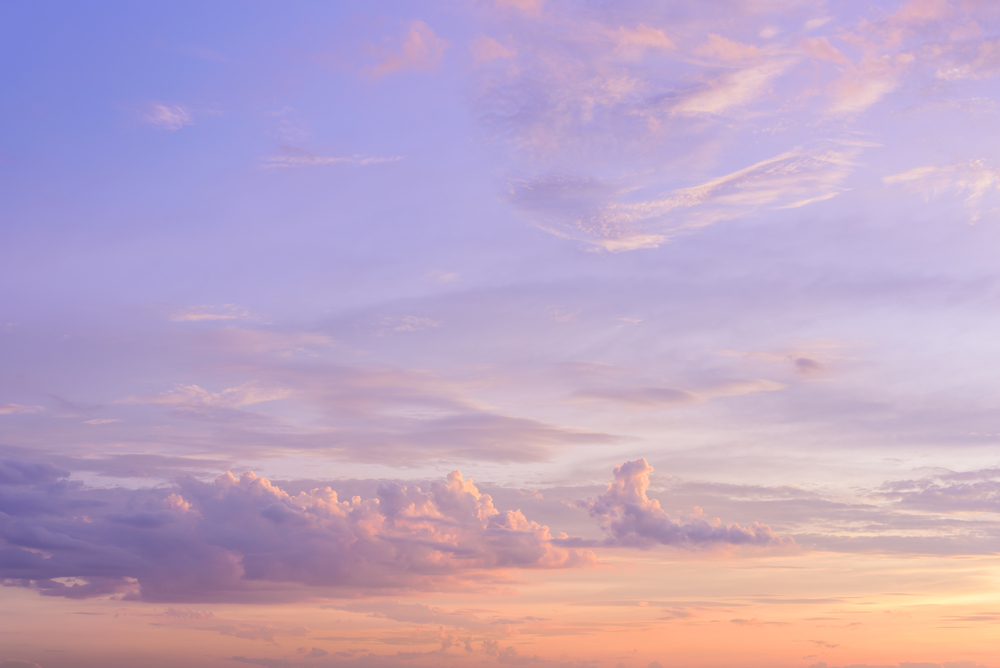
When it comes to predicting the impact that machine learning is likely to have on society, it can be difficult to separate hyperbole from reality. AI will either be humanity’s savior or the cause of its demise. Sam Altman, CEO of OpenAI (the company behind ChatGPT), once said, "A.I. will probably most likely lead to the end of the world, but in the meantime, there'll be great companies."
While we certainly hope that the apocalyptic scenario never materializes, there is no questioning the second half of Altman’s assertion. AI is already transforming numerous business sectors, including weather forecasting. In this context, Salient Predictions has introduced AI into S2S and long-range weather forecasting - from two weeks to one year.
AI is revolutionizing weather forecasting
Historically, weather forecasting has heavily relied on numerical weather prediction (NWP) models. These models, grounded in established physical laws, simulate atmospheric and oceanic processes with considerable detail and precision. This involves numerically solving complex differential equations on a global scale using supercomputers - a process that requires the financial and human resources available only to governmental organizations.
AI models use a fundamentally different approach. Instead of starting with the underlying laws of physics, AI models consume vast amounts of data and learn to extract patterns that describe these data. Specifically, when applied to the problem of weather forecasting, AI models are in principle capable of learning the underlying physics purely from data. This is an improvement because physical models are limited in their capacity to capture all relevant physical processes and to properly quantify the uncertainty of the forecast. Additionally, AI models are better able to identify specific conditions within the climate system that yield more accurate forecasts – forecasts that diverge from the forecasts of traditional models – called 'forecasts of opportunity.' Another strength of AI models is their efficiency. Leveraging the power of GPU-accelerated computing, they are significantly more cost-effective and faster to develop and operate. Tasks previously requiring a large scientific team and access to supercomputers can now be accomplished with a few GPUs and a lean team. Importantly, once the AI models are trained using historical data, the incremental cost of generating new forecasts becomes almost negligible. As a result, an increasing number of companies are directing their investments toward AI weather models.
However, much of the focus remains on short- to medium-range forecasts (up to ten days), a timescale where physical models already perform exceptionally well. In contrast, Salient is pioneering advancements in the less-developed timescale of S2S forecasting.
S2S is different
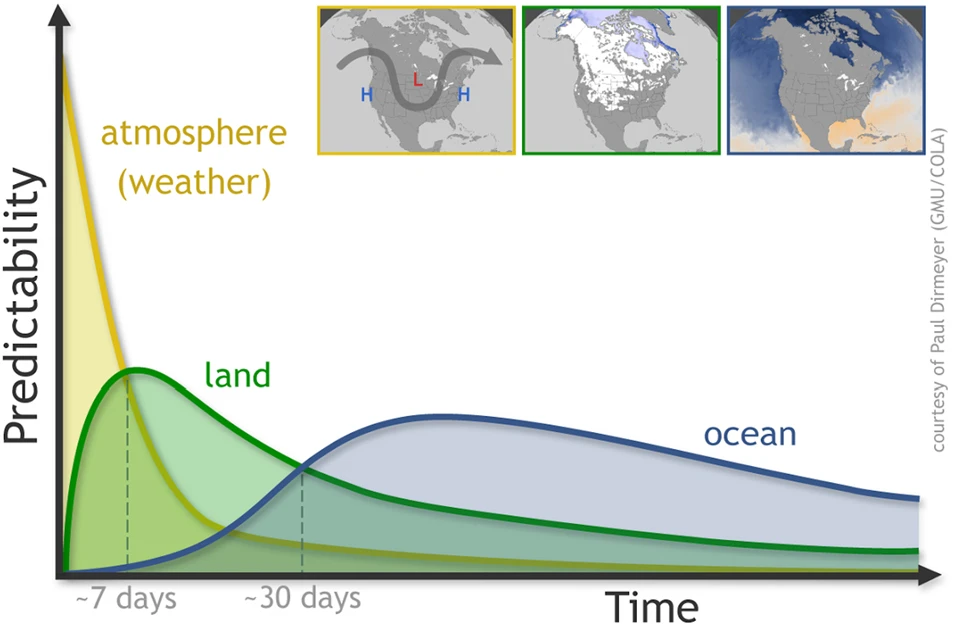
S2S forecasting deals with the longer-term, non-linear interaction between the atmosphere, oceans, and land surface. This includes factors like ocean heat content, snow cover, and soil moisture, which can influence the atmosphere over weeks and months. Delivering accurate predictions at these timescales is significantly more challenging from a physics perspective due to the increasing complexity and chaos inherent in the climate system.
Therefore, adopting a probabilistic approach becomes critical. Instead of predicting an exact temperature for a specific day or week in the future, for example, we assign a probability to a range of potential outcomes. S2S forecasting is not about getting every single forecast exactly right (the laws of physics preclude that). Instead, it is about building models that, over a range of forecasts, offer an edge over simple baselines such as climatology or persistence forecasts.
To draw an analogy, consider the game of poker. Expecting consistent wins in poker is unrealistic due to the significant role of chance. Still, there are easily measurable disparities in player abilities. Similarly, access to improved probabilistic S2S forecasts can significantly improve decision-making in business. Companies that routinely make weather-related decisions over extended timescales and diverse geographic locations — like those in the agriculture, energy, and commodity trading sectors — can benefit from these improvements. And while each individual improvement might seem small, their cumulative effect can significantly enhance the company's bottom line over time.
What specific improvements do AI methods bring to S2S forecasting? First, NWP models sometimes fall short in capturing specific non-linear interactions that can yield large-scale effects over extended timescales. For example, deep learning methods can more easily pick up on long-range spatio-temporal teleconnections in the climate system, such as the connection between ocean salinity and increased precipitation over land. This offers a distinct advantage in long-range forecasting. Second, traditional NWP models, despite providing ensemble forecasts, can grapple with forecast bias and underestimation of uncertainty. A forecast for a particular outcome with, say, a 70% probability, may not happen 70% of the time. Natively probabilistic AI models, such as those we are developing at Salient, tackle these limitations by providing more reliable and calibrated estimates of forecast uncertainty, which can help users make better-informed decisions
Finding the right formula at Salient
Given the potential for machine learning to improve S2S forecasting accuracy, why aren’t more forecasters leveraging it? Building accurate and useful AI S2S forecasting models requires getting three things right. First, it requires a multidisciplinary team of machine learning and climate modeling experts. Second, it is important to establish a robust testing and quality control process for the models. Finally, it requires creating and maintaining a cloud-based infrastructure capable of handling large-scale data analysis and facilitating model experimentation.
At Salient, we are proud to have brought these things together for the benefit of our customers who are leveraging Salient’s forecasts to reduce cost and improve resilience across a range of industries - energy, finance, agriculture, supply chain, and beyond.
October 15, 2023
October 15, 2023
Machine Learning Offers New Possibilities in S2S Forecasting
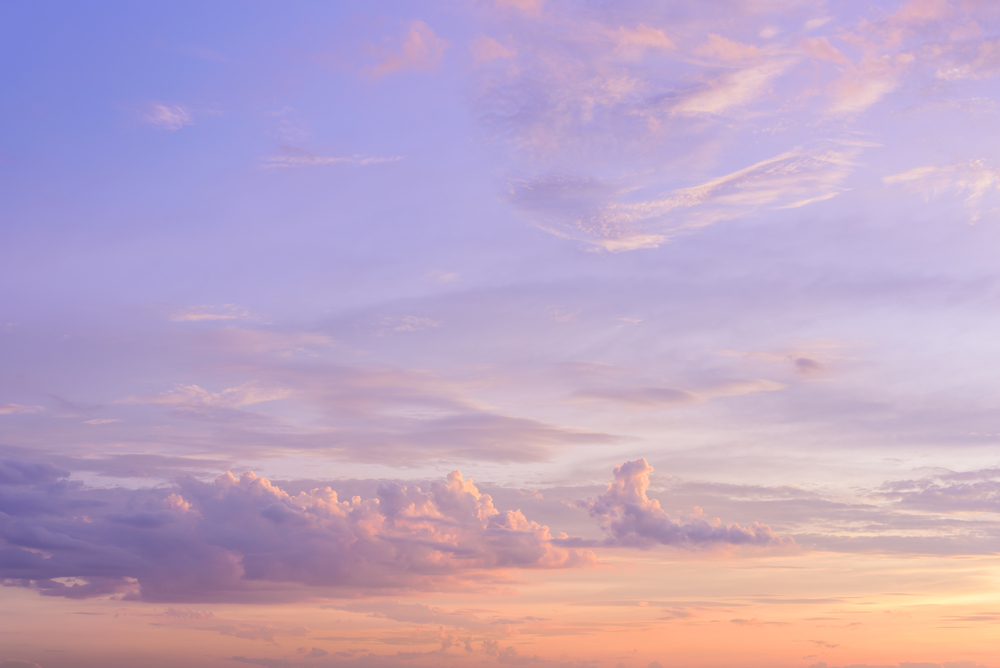
When it comes to predicting the impact that machine learning is likely to have on society, it can be difficult to separate hyperbole from reality. AI will either be humanity’s savior or the cause of its demise. Sam Altman, CEO of OpenAI (the company behind ChatGPT), once said, "A.I. will probably most likely lead to the end of the world, but in the meantime, there'll be great companies."
While we certainly hope that the apocalyptic scenario never materializes, there is no questioning the second half of Altman’s assertion. AI is already transforming numerous business sectors, including weather forecasting. In this context, Salient Predictions has introduced AI into S2S and long-range weather forecasting - from two weeks to one year.
AI is revolutionizing weather forecasting
Historically, weather forecasting has heavily relied on numerical weather prediction (NWP) models. These models, grounded in established physical laws, simulate atmospheric and oceanic processes with considerable detail and precision. This involves numerically solving complex differential equations on a global scale using supercomputers - a process that requires the financial and human resources available only to governmental organizations.
AI models use a fundamentally different approach. Instead of starting with the underlying laws of physics, AI models consume vast amounts of data and learn to extract patterns that describe these data. Specifically, when applied to the problem of weather forecasting, AI models are in principle capable of learning the underlying physics purely from data. This is an improvement because physical models are limited in their capacity to capture all relevant physical processes and to properly quantify the uncertainty of the forecast. Additionally, AI models are better able to identify specific conditions within the climate system that yield more accurate forecasts – forecasts that diverge from the forecasts of traditional models – called 'forecasts of opportunity.' Another strength of AI models is their efficiency. Leveraging the power of GPU-accelerated computing, they are significantly more cost-effective and faster to develop and operate. Tasks previously requiring a large scientific team and access to supercomputers can now be accomplished with a few GPUs and a lean team. Importantly, once the AI models are trained using historical data, the incremental cost of generating new forecasts becomes almost negligible. As a result, an increasing number of companies are directing their investments toward AI weather models.
However, much of the focus remains on short- to medium-range forecasts (up to ten days), a timescale where physical models already perform exceptionally well. In contrast, Salient is pioneering advancements in the less-developed timescale of S2S forecasting.
S2S is different
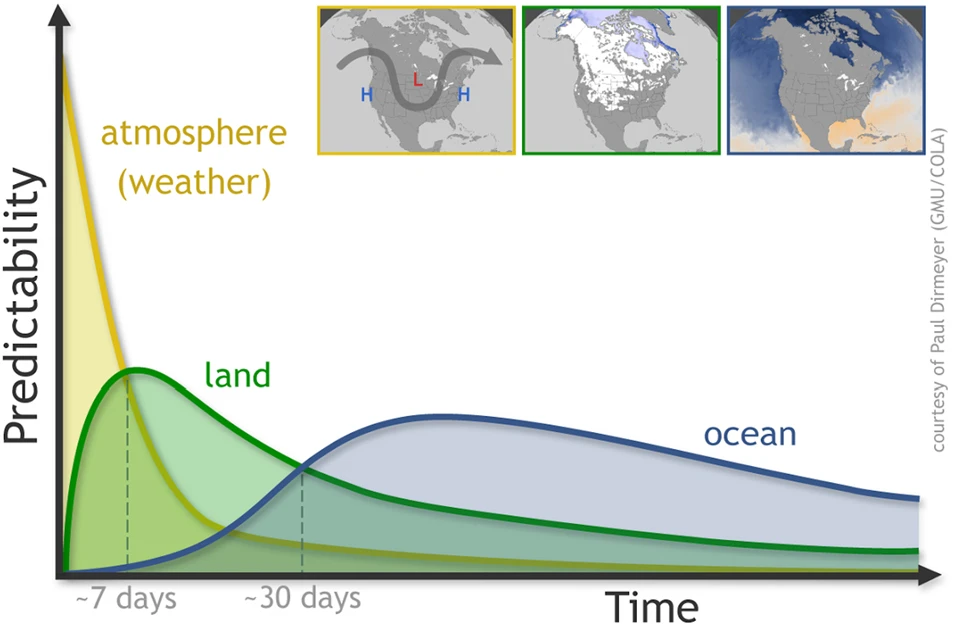
S2S forecasting deals with the longer-term, non-linear interaction between the atmosphere, oceans, and land surface. This includes factors like ocean heat content, snow cover, and soil moisture, which can influence the atmosphere over weeks and months. Delivering accurate predictions at these timescales is significantly more challenging from a physics perspective due to the increasing complexity and chaos inherent in the climate system.
Therefore, adopting a probabilistic approach becomes critical. Instead of predicting an exact temperature for a specific day or week in the future, for example, we assign a probability to a range of potential outcomes. S2S forecasting is not about getting every single forecast exactly right (the laws of physics preclude that). Instead, it is about building models that, over a range of forecasts, offer an edge over simple baselines such as climatology or persistence forecasts.
To draw an analogy, consider the game of poker. Expecting consistent wins in poker is unrealistic due to the significant role of chance. Still, there are easily measurable disparities in player abilities. Similarly, access to improved probabilistic S2S forecasts can significantly improve decision-making in business. Companies that routinely make weather-related decisions over extended timescales and diverse geographic locations — like those in the agriculture, energy, and commodity trading sectors — can benefit from these improvements. And while each individual improvement might seem small, their cumulative effect can significantly enhance the company's bottom line over time.
What specific improvements do AI methods bring to S2S forecasting? First, NWP models sometimes fall short in capturing specific non-linear interactions that can yield large-scale effects over extended timescales. For example, deep learning methods can more easily pick up on long-range spatio-temporal teleconnections in the climate system, such as the connection between ocean salinity and increased precipitation over land. This offers a distinct advantage in long-range forecasting. Second, traditional NWP models, despite providing ensemble forecasts, can grapple with forecast bias and underestimation of uncertainty. A forecast for a particular outcome with, say, a 70% probability, may not happen 70% of the time. Natively probabilistic AI models, such as those we are developing at Salient, tackle these limitations by providing more reliable and calibrated estimates of forecast uncertainty, which can help users make better-informed decisions
Finding the right formula at Salient
Given the potential for machine learning to improve S2S forecasting accuracy, why aren’t more forecasters leveraging it? Building accurate and useful AI S2S forecasting models requires getting three things right. First, it requires a multidisciplinary team of machine learning and climate modeling experts. Second, it is important to establish a robust testing and quality control process for the models. Finally, it requires creating and maintaining a cloud-based infrastructure capable of handling large-scale data analysis and facilitating model experimentation.
At Salient, we are proud to have brought these things together for the benefit of our customers who are leveraging Salient’s forecasts to reduce cost and improve resilience across a range of industries - energy, finance, agriculture, supply chain, and beyond.
About Salient
Salient combines ocean and land-surface data with machine learning and climate expertise to deliver accurate and reliable subseasonal-to-seasonal weather forecasts and industry insights—two to 52 weeks in advance. Bringing together leading experts in physical oceanography, climatology and the global water cycle, machine learning, and AI, Salient helps enterprise clients improve resiliency, increase preparedness, and make better decisions in the face of a rapidly changing climate. Learn more at www.salientpredictions.com and follow on LinkedIn and X.