
January 17, 2025
January 16, 2025
The Benefits of Large Ensembles for Subseasonal Forecasting
Summary: Ensemble forecasts with subseasonal forecasting systems enables forecasters to assess the probability and confidence in subseasonal forecasts. The Salient team will soon launch a new version of their forecasting model - version 10, coming in Q1 2025 - which will have the capability of producing 1000+ ensemble members per forecast run, which is 20-100x more members than comparable dynamical models. The advantages of having large ensembles include:
- more robust forecasts
- improved prediction of extreme events, and
- furthering the science of subseasonal forecasting
The bottom line? Better support for decision-makers across multiple sectors through more reliable and trustworthy forecasts from a data-driven model.

Why Ensemble Forecasting?
Subseasonal-to-seasonal (S2S) forecasting, unlike shorter-range forecasting, relies heavily on a probabilistic approach to forecasting - i.e., providing a range of potential forecasts for fields of interest (e.g., temperature, rainfall, wind, cloud cover). Probabilistic forecasts play a critical role in sectors like agriculture (example), energy (example; example), and water resource management. To find out more about harnessing probabilistic forecasts for such decisions, you can watch this Salient webinar in which I participated in April 2024.
The image below demonstrates the basic idea of ensemble forecasting and how we can use the ensembles to quantify the uncertainty (i.e., range of error) in the forecast.
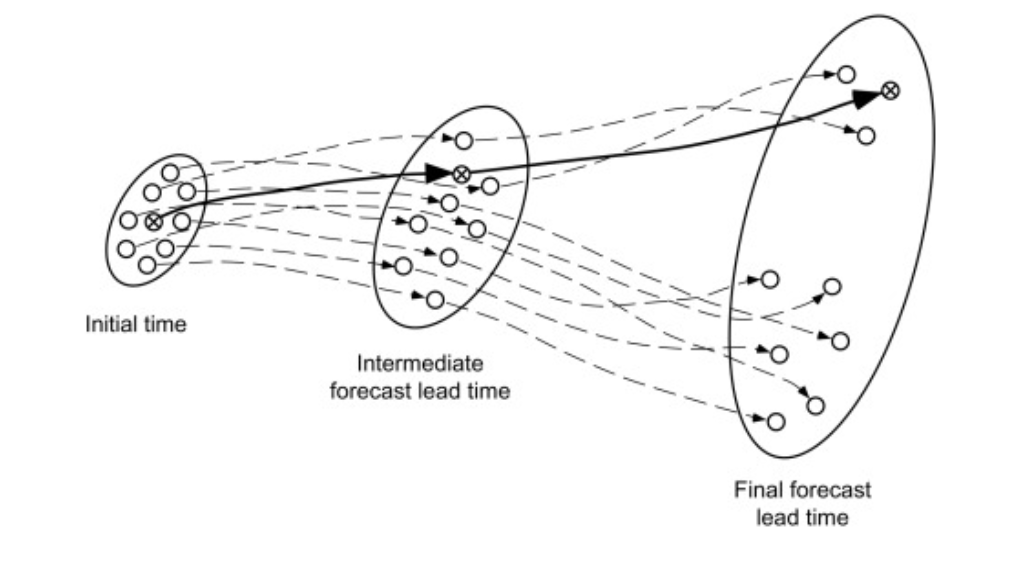
In short, with data-driven subseasonal forecasting models like those used by Salient, the number of ensemble members—i.e., independent model runs with slightly different initial conditions—can significantly influence our view of a forecast and shape our confidence and understanding of what to expect on the subseasonal timescale.
Referring to the image above, in order to better represent the potential distribution of subseasonal weather events, especially extreme events, and thus reduce uncertainty in the forecasts, we would like many open circles in the far-right oval. Unfortunately, this is difficult to do with conventional subseasonal forecast models. Global (and high-resolution regional) dynamical forecast models (i.e., weather models which solve physics-based equations over time) are computationally expensive to run just a single run, so major centers like ECMWF and NOAA NCEP are limited to how many ensemble members can be run per forecast cycle.
But the good news is Salient’s team of scientists are working on the latest version of their subseasonal model (version 10) that, along with updated outputs and improved statistical / machine-learning methods, will also have the capability to produce 1000+ ensemble members for each run! This is an incredible feat and highly beneficial for the subseasonal forecasting community and its users.
Here are three (3) major advantages of ensemble forecasting for subseasonal forecasts and how Salient’s v10 model may be a game-changer in this forecasting space.
1. Better-Defined Probability Distributions and Improved Robustness of Forecasts
Ensemble forecasting enables forecasters to better quantify uncertainty and provide more actionable probabilistic forecasts for decision-makers simply because we have a larger sample size (n) of potential future weather states. Indeed, as the number of (independent) samples increases, the probability distribution of the mean of a variable resembles more like a normal distribution, no matter what the original distribution of that variable was. Furthermore, the spread (or uncertainty) for that variable’s distribution is proportional to n-1/2 (i.e., higher n, smaller spread or uncertainty). Thus, for practitioners, sample size is a big deal when it comes to confidence in conclusions drawn about the occurrence of an event.
Consider two subseasonal forecast models identical in every way except Model #1 has 10 ensemble members and Model #2 has 1000 ensemble members. In Model #1, if 8 ensemble members predict rainfall exceeding a certain threshold over a given region during Week 4, that would mean there is an 80% probability of the event occurring (i.e., 8 out of 10 members). In Model #2, if 800 ensemble members forecast the same extreme rainfall event over the same region, that too represents an 80% probability of the extreme event occurring (i.e., 800 out of 1000 members). But the difference in our assessment of confidence in the likelihood of the event occurring is very different – i.e., confidence of the event occurring is much greater with Model #2 since we sampled 100 times more possible futures!
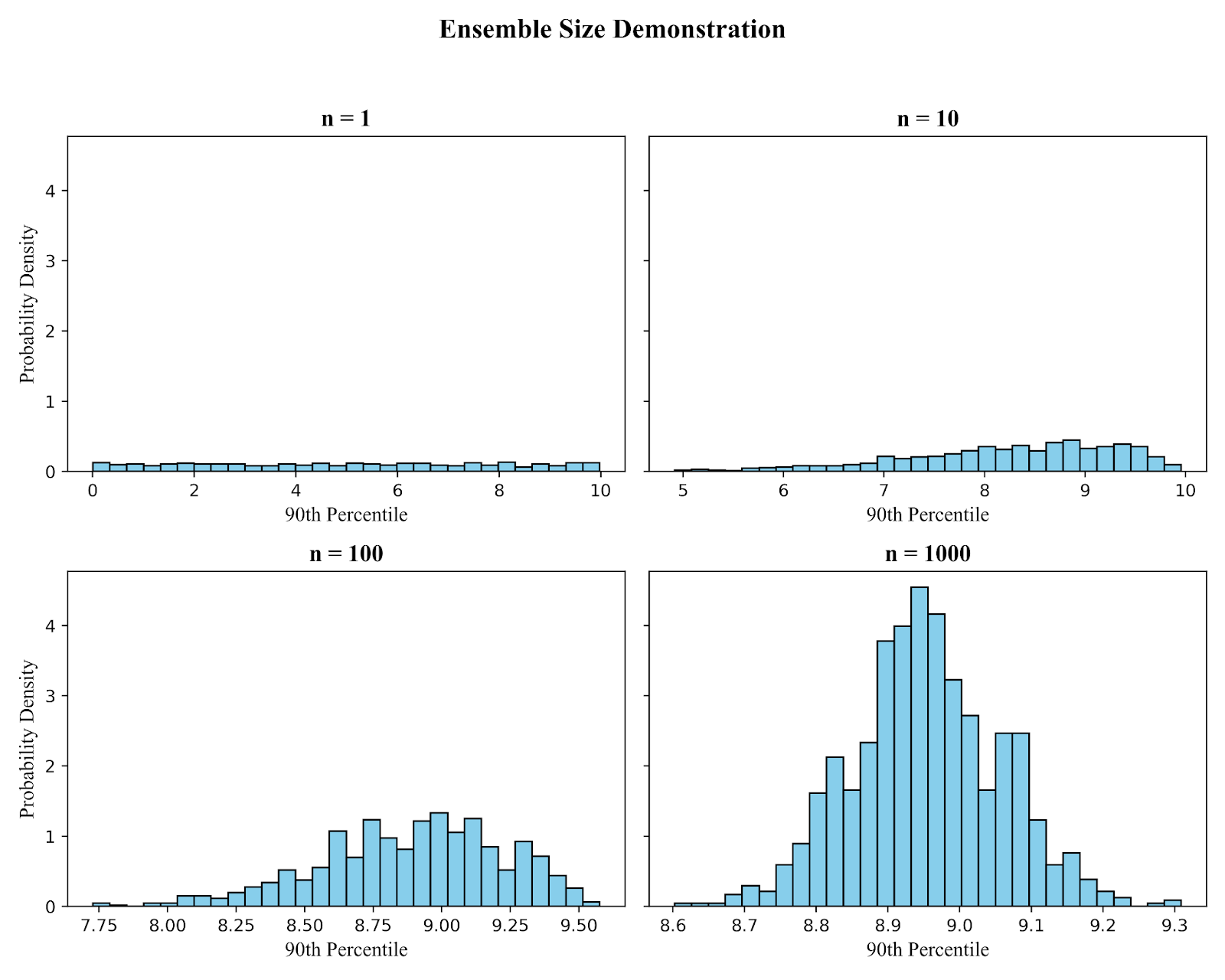
Additionally, models with more ensemble members mitigate the impact of outliers or errors from any single member. Indeed, by averaging across a large number of members, the ensemble mean becomes a more reliable representation of the expected outcome (i.e., see the image above). Indeed, larger ensemble sizes enhance the granularity and reliability of probabilistic forecasts, providing end users with actionable insights tailored to their specific needs. This robustness is particularly beneficial in subseasonal forecasting, where individual model runs may struggle to capture the full range of possible weather patterns. Larger ensembles can help counteract the impact of biases on the forecast by averaging across multiple model outputs, each with slightly different characteristics. In essence, such averaging can "smooth out" the noise and amplify the signal, leading to more consistent, accurate, and reliable forecasts.
2. Improved Skill in Extreme Event Prediction on Subseasonal Timescales
Predicting extreme weather events such as heatwaves, cold spells, or extreme rainfall is a major challenge for subseasonal forecasting. These events are rare by nature, making it difficult for individual model runs to capture their occurrence accurately and more reliably. This fact is even true for data-driven forecast models, as these models rely on deriving signals from the observational record. As such, it is even more important for dynamical and data-driven models to have a larger number of ensemble members to provide a broader sampling of the potential state space, increasing the likelihood that some members will successfully predict extreme conditions.
This advantage of ensemble forecasting is especially critical in subseasonal forecasting, since many decision-makers across multiple sectors are most interested in the potential for temperature and precipitation extremes. For instance, early identification of a potential heatwave can help public health agencies and energy providers prepare for increased demand and mitigate risks to vulnerable populations. There are examples of this “large ensemble, extreme weather prediction” approach already underway by government agencies, academia, and private industry. Salient’s new model’s capability of 1000s of ensemble members will be a huge benefit in this effort for science and for its customers.
Such improved skill for extreme events also translates to another facet for the Salient v10 model: the development of large-ensemble hindcasts. The term “hindcast” or “reforecast” refers to runs of a forecast model that are produced for the past, using information that was available at the time of the start of the run. Hindcasts have several uses including scoring model skill and assessing model biases. Additionally, large ensemble hindcasts allow for exploring and characterizing observed extreme weather events much better. This is very important because, by definition, the historical record is limited, especially for extreme events. Such an approach has already been used with dynamical model hindcast archives in real world situations - e.g., the potential return period of heat extremes in South Africa and assessing the potential impact of extreme rain events on water quality in New York City. With a hindcast archive of many ensemble members, Salient’s team will be well-positioned to answer key client questions concerning how far in advance a reliable, accurate forecast of an extreme event is expected.
3. Advancing the Science Behind Subseasonal Forecasting and the Earth Climate System
Having a large ensemble size allows scientists to explore a wider range of potential physical states and interactions within the Earth system. This diversity in the potential states of the climate system is especially valuable for data-driven models, which often rely on statistical representations of physical processes. Indeed, a huge barrier for the acceptance of data-driven forecasts on the subseasonal timescale is trust of such model forecasts, particularly in the interpretability or explainability of these so-called “black box models.” By incorporating multiple ensemble members into the forecasting process, data-driven models have more opportunities to capture subtle and/or other nonlinear interactions that might be missed in a single run. This idea is especially relevant for key drivers of subseasonal forecasts such as the Madden-Julian Oscillation, ocean-atmosphere interactions, and other teleconnection patterns.
Large ensembles in subseasonal forecasting also enable researchers to test hypotheses about the Earth system, including exploring how sensitive forecasts are to initial conditions or to the evolution of specific physical processes. For example, in a study done in my research lab, we explored 51 ensemble members of the ECMWF subseasonal forecasting system to show that the accuracy of long-lead (3 weeks out) forecasts of the February 2021 North American cold air outbreak strongly relied on whether if an ensemble member correctly captured a key atmospheric “wave break” in the Atlantic ahead of the event. Those ensemble members that captured this wave break had a much better forecast (albeit not perfect) of the February 2021 cold wave, while ensemble members that did not forecast the wave break actually forecasted above normal temperatures for the Central US in February 2021. Such tests and inquiries would be even more valuable with 1000+ ensemble members for other events and allow the scientific community to gain more insight into what atmospheric and/or oceanic phenomena are key drivers for extreme weather events.
Key takeaway: Improving the scientific knowledge and rigor behind a subseasonal forecasting system drives more confidence and trust in its forecasts.
Conclusion
In the quest for more accurate and more reliable subseasonal forecasts, the use of large ensemble sizes in data-driven models has emerged as a game-changer. From reducing uncertainty and improving robustness to enhancing extreme event prediction and supporting actionable scientifically-based decisions, the benefits of this approach may be transformative. As computational capabilities continue to grow and machine-learning techniques evolve, the potential of large ensembles will only increase in the subseasonal forecasting space. Salient’s team of scientists is at the forefront of this emerging paradigm, and the release of the Salient v10 model should provide a remarkable step toward more reliable subseasonal forecasts.
January 17, 2025
January 16, 2025
The Benefits of Large Ensembles for Subseasonal Forecasting
Summary: Ensemble forecasts with subseasonal forecasting systems enables forecasters to assess the probability and confidence in subseasonal forecasts. The Salient team will soon launch a new version of their forecasting model - version 10, coming in Q1 2025 - which will have the capability of producing 1000+ ensemble members per forecast run, which is 20-100x more members than comparable dynamical models. The advantages of having large ensembles include:
- more robust forecasts
- improved prediction of extreme events, and
- furthering the science of subseasonal forecasting
The bottom line? Better support for decision-makers across multiple sectors through more reliable and trustworthy forecasts from a data-driven model.

Why Ensemble Forecasting?
Subseasonal-to-seasonal (S2S) forecasting, unlike shorter-range forecasting, relies heavily on a probabilistic approach to forecasting - i.e., providing a range of potential forecasts for fields of interest (e.g., temperature, rainfall, wind, cloud cover). Probabilistic forecasts play a critical role in sectors like agriculture (example), energy (example; example), and water resource management. To find out more about harnessing probabilistic forecasts for such decisions, you can watch this Salient webinar in which I participated in April 2024.
The image below demonstrates the basic idea of ensemble forecasting and how we can use the ensembles to quantify the uncertainty (i.e., range of error) in the forecast.
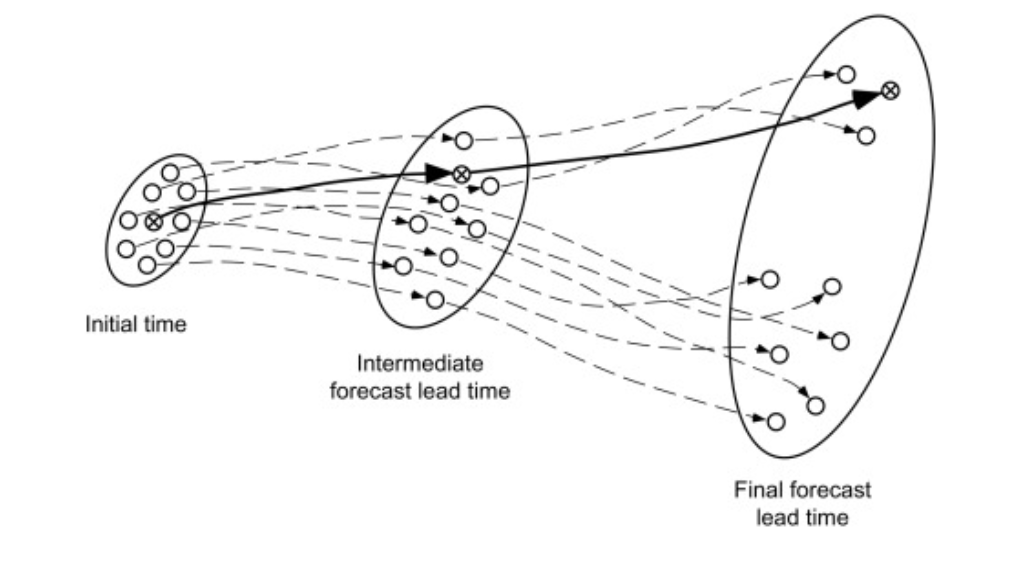
In short, with data-driven subseasonal forecasting models like those used by Salient, the number of ensemble members—i.e., independent model runs with slightly different initial conditions—can significantly influence our view of a forecast and shape our confidence and understanding of what to expect on the subseasonal timescale.
Referring to the image above, in order to better represent the potential distribution of subseasonal weather events, especially extreme events, and thus reduce uncertainty in the forecasts, we would like many open circles in the far-right oval. Unfortunately, this is difficult to do with conventional subseasonal forecast models. Global (and high-resolution regional) dynamical forecast models (i.e., weather models which solve physics-based equations over time) are computationally expensive to run just a single run, so major centers like ECMWF and NOAA NCEP are limited to how many ensemble members can be run per forecast cycle.
But the good news is Salient’s team of scientists are working on the latest version of their subseasonal model (version 10) that, along with updated outputs and improved statistical / machine-learning methods, will also have the capability to produce 1000+ ensemble members for each run! This is an incredible feat and highly beneficial for the subseasonal forecasting community and its users.
Here are three (3) major advantages of ensemble forecasting for subseasonal forecasts and how Salient’s v10 model may be a game-changer in this forecasting space.
1. Better-Defined Probability Distributions and Improved Robustness of Forecasts
Ensemble forecasting enables forecasters to better quantify uncertainty and provide more actionable probabilistic forecasts for decision-makers simply because we have a larger sample size (n) of potential future weather states. Indeed, as the number of (independent) samples increases, the probability distribution of the mean of a variable resembles more like a normal distribution, no matter what the original distribution of that variable was. Furthermore, the spread (or uncertainty) for that variable’s distribution is proportional to n-1/2 (i.e., higher n, smaller spread or uncertainty). Thus, for practitioners, sample size is a big deal when it comes to confidence in conclusions drawn about the occurrence of an event.
Consider two subseasonal forecast models identical in every way except Model #1 has 10 ensemble members and Model #2 has 1000 ensemble members. In Model #1, if 8 ensemble members predict rainfall exceeding a certain threshold over a given region during Week 4, that would mean there is an 80% probability of the event occurring (i.e., 8 out of 10 members). In Model #2, if 800 ensemble members forecast the same extreme rainfall event over the same region, that too represents an 80% probability of the extreme event occurring (i.e., 800 out of 1000 members). But the difference in our assessment of confidence in the likelihood of the event occurring is very different – i.e., confidence of the event occurring is much greater with Model #2 since we sampled 100 times more possible futures!
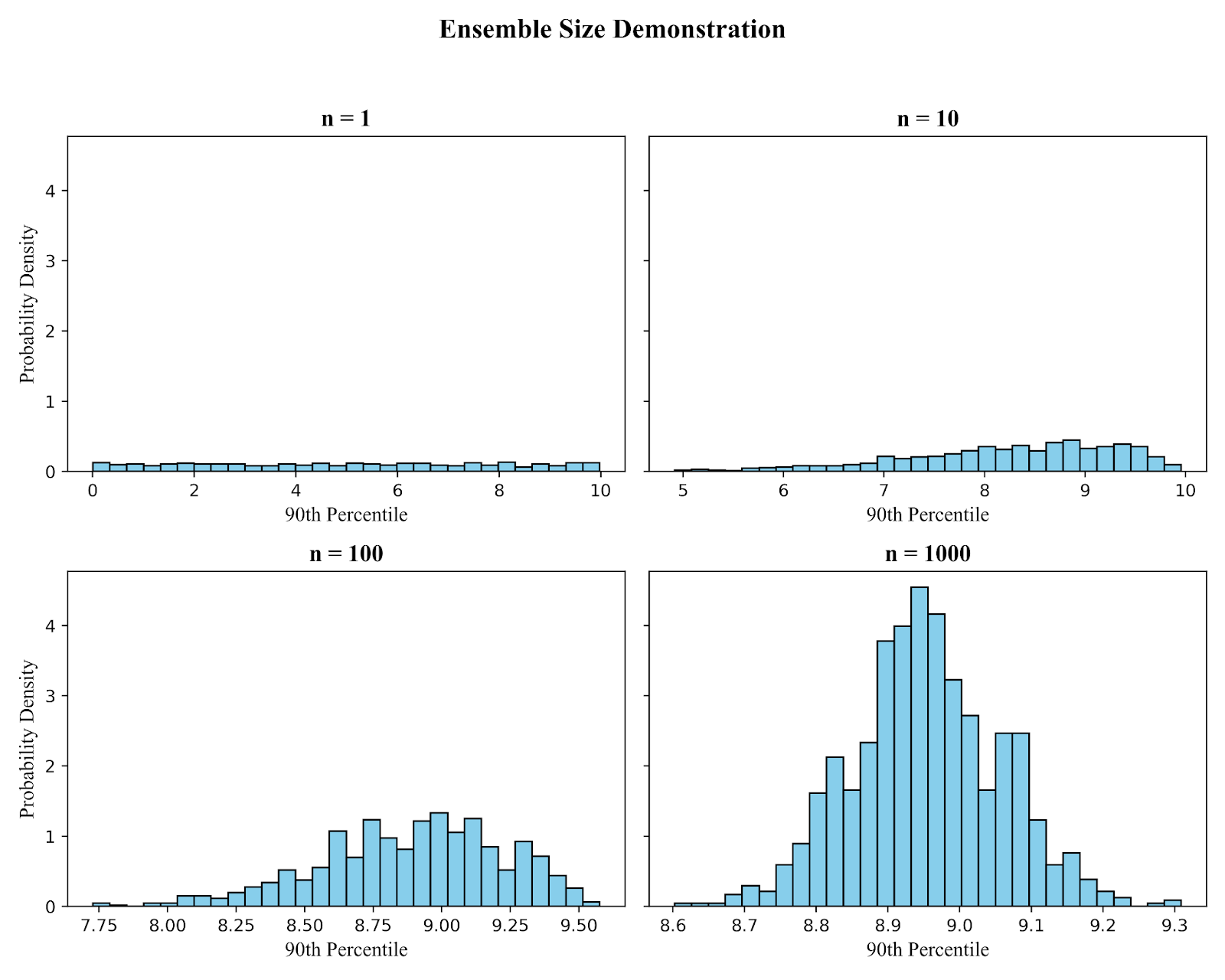
Additionally, models with more ensemble members mitigate the impact of outliers or errors from any single member. Indeed, by averaging across a large number of members, the ensemble mean becomes a more reliable representation of the expected outcome (i.e., see the image above). Indeed, larger ensemble sizes enhance the granularity and reliability of probabilistic forecasts, providing end users with actionable insights tailored to their specific needs. This robustness is particularly beneficial in subseasonal forecasting, where individual model runs may struggle to capture the full range of possible weather patterns. Larger ensembles can help counteract the impact of biases on the forecast by averaging across multiple model outputs, each with slightly different characteristics. In essence, such averaging can "smooth out" the noise and amplify the signal, leading to more consistent, accurate, and reliable forecasts.
2. Improved Skill in Extreme Event Prediction on Subseasonal Timescales
Predicting extreme weather events such as heatwaves, cold spells, or extreme rainfall is a major challenge for subseasonal forecasting. These events are rare by nature, making it difficult for individual model runs to capture their occurrence accurately and more reliably. This fact is even true for data-driven forecast models, as these models rely on deriving signals from the observational record. As such, it is even more important for dynamical and data-driven models to have a larger number of ensemble members to provide a broader sampling of the potential state space, increasing the likelihood that some members will successfully predict extreme conditions.
This advantage of ensemble forecasting is especially critical in subseasonal forecasting, since many decision-makers across multiple sectors are most interested in the potential for temperature and precipitation extremes. For instance, early identification of a potential heatwave can help public health agencies and energy providers prepare for increased demand and mitigate risks to vulnerable populations. There are examples of this “large ensemble, extreme weather prediction” approach already underway by government agencies, academia, and private industry. Salient’s new model’s capability of 1000s of ensemble members will be a huge benefit in this effort for science and for its customers.
Such improved skill for extreme events also translates to another facet for the Salient v10 model: the development of large-ensemble hindcasts. The term “hindcast” or “reforecast” refers to runs of a forecast model that are produced for the past, using information that was available at the time of the start of the run. Hindcasts have several uses including scoring model skill and assessing model biases. Additionally, large ensemble hindcasts allow for exploring and characterizing observed extreme weather events much better. This is very important because, by definition, the historical record is limited, especially for extreme events. Such an approach has already been used with dynamical model hindcast archives in real world situations - e.g., the potential return period of heat extremes in South Africa and assessing the potential impact of extreme rain events on water quality in New York City. With a hindcast archive of many ensemble members, Salient’s team will be well-positioned to answer key client questions concerning how far in advance a reliable, accurate forecast of an extreme event is expected.
3. Advancing the Science Behind Subseasonal Forecasting and the Earth Climate System
Having a large ensemble size allows scientists to explore a wider range of potential physical states and interactions within the Earth system. This diversity in the potential states of the climate system is especially valuable for data-driven models, which often rely on statistical representations of physical processes. Indeed, a huge barrier for the acceptance of data-driven forecasts on the subseasonal timescale is trust of such model forecasts, particularly in the interpretability or explainability of these so-called “black box models.” By incorporating multiple ensemble members into the forecasting process, data-driven models have more opportunities to capture subtle and/or other nonlinear interactions that might be missed in a single run. This idea is especially relevant for key drivers of subseasonal forecasts such as the Madden-Julian Oscillation, ocean-atmosphere interactions, and other teleconnection patterns.
Large ensembles in subseasonal forecasting also enable researchers to test hypotheses about the Earth system, including exploring how sensitive forecasts are to initial conditions or to the evolution of specific physical processes. For example, in a study done in my research lab, we explored 51 ensemble members of the ECMWF subseasonal forecasting system to show that the accuracy of long-lead (3 weeks out) forecasts of the February 2021 North American cold air outbreak strongly relied on whether if an ensemble member correctly captured a key atmospheric “wave break” in the Atlantic ahead of the event. Those ensemble members that captured this wave break had a much better forecast (albeit not perfect) of the February 2021 cold wave, while ensemble members that did not forecast the wave break actually forecasted above normal temperatures for the Central US in February 2021. Such tests and inquiries would be even more valuable with 1000+ ensemble members for other events and allow the scientific community to gain more insight into what atmospheric and/or oceanic phenomena are key drivers for extreme weather events.
Key takeaway: Improving the scientific knowledge and rigor behind a subseasonal forecasting system drives more confidence and trust in its forecasts.
Conclusion
In the quest for more accurate and more reliable subseasonal forecasts, the use of large ensemble sizes in data-driven models has emerged as a game-changer. From reducing uncertainty and improving robustness to enhancing extreme event prediction and supporting actionable scientifically-based decisions, the benefits of this approach may be transformative. As computational capabilities continue to grow and machine-learning techniques evolve, the potential of large ensembles will only increase in the subseasonal forecasting space. Salient’s team of scientists is at the forefront of this emerging paradigm, and the release of the Salient v10 model should provide a remarkable step toward more reliable subseasonal forecasts.
About Salient
Salient combines ocean and land-surface data with machine learning and climate expertise to deliver accurate and reliable subseasonal-to-seasonal weather forecasts and industry insights—two to 52 weeks in advance. Bringing together leading experts in physical oceanography, climatology and the global water cycle, machine learning, and AI, Salient helps enterprise clients improve resiliency, increase preparedness, and make better decisions in the face of a rapidly changing climate. Learn more at www.salientpredictions.com and follow on LinkedIn and X.