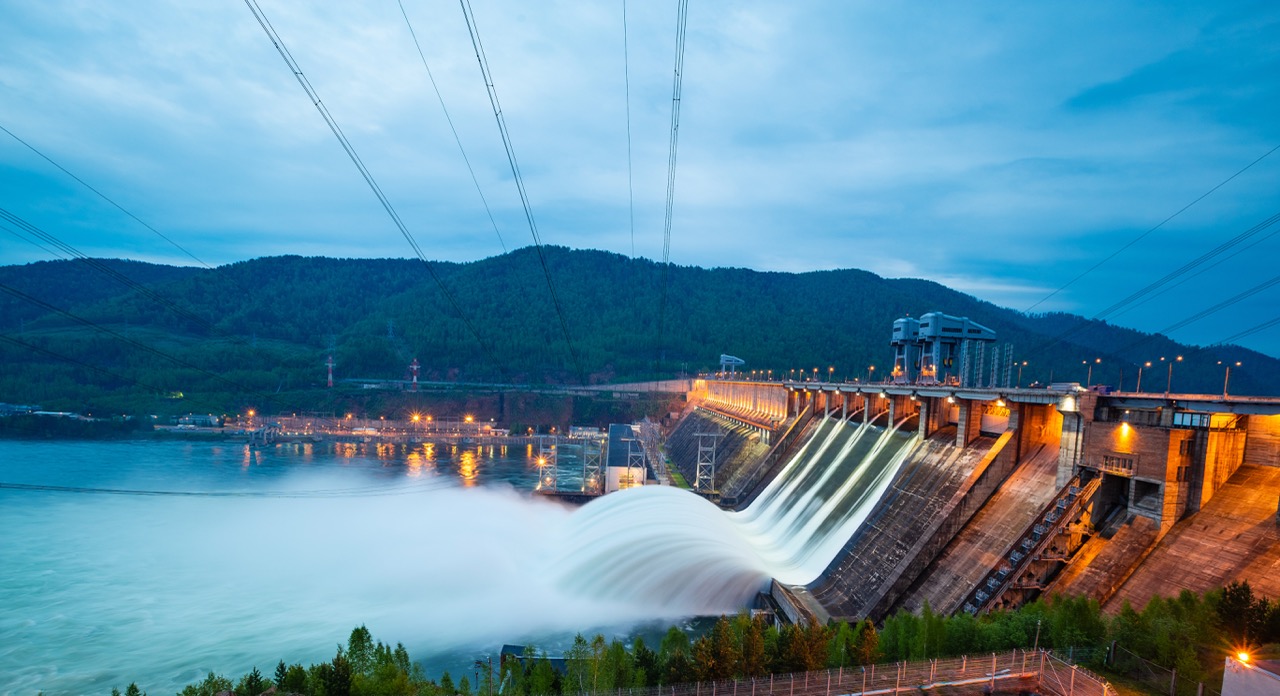
March 15, 2024
March 15, 2024
The Challenge & Importance of Forecasting Sudden Stratospheric Warming Events
Summary: Salient’s AI-powered methodology reduces bias in forecasting Sudden Stratospheric Warming (SSW) events, which are difficult to predict using physics-based models and important because they affect weather at the earth’s surface.

Introduction to Sudden Stratospheric Warming Events
The stratosphere begins 10 km above the surface; how can weather conditions so far from the surface impact weather on the ground? Research over the past 20 years has revealed that certain conditions can disrupt the stratospheric polar vortex – and this can indeed impact winter weather at Earth’s surface. Recently, research into the predictability of SSW events and their associated surface impacts has accelerated.
The polar vortex, a ring of strong winds circling the polar regions, can weaken and even split into two during Sudden Stratospheric Warming (SSW) events, pushing cold air south. Although challenging to predict, these occurrences can have significant impacts on global surface weather conditions. The statistically significant effects of SSW events (Figure 1) are most pronounced in northern Siberia, leading to colder temperatures, while Western Europe often experiences increased precipitation. Also, SSW events on average have a small but significant impact on the eastern United States, leading to colder-than-usual temperatures.
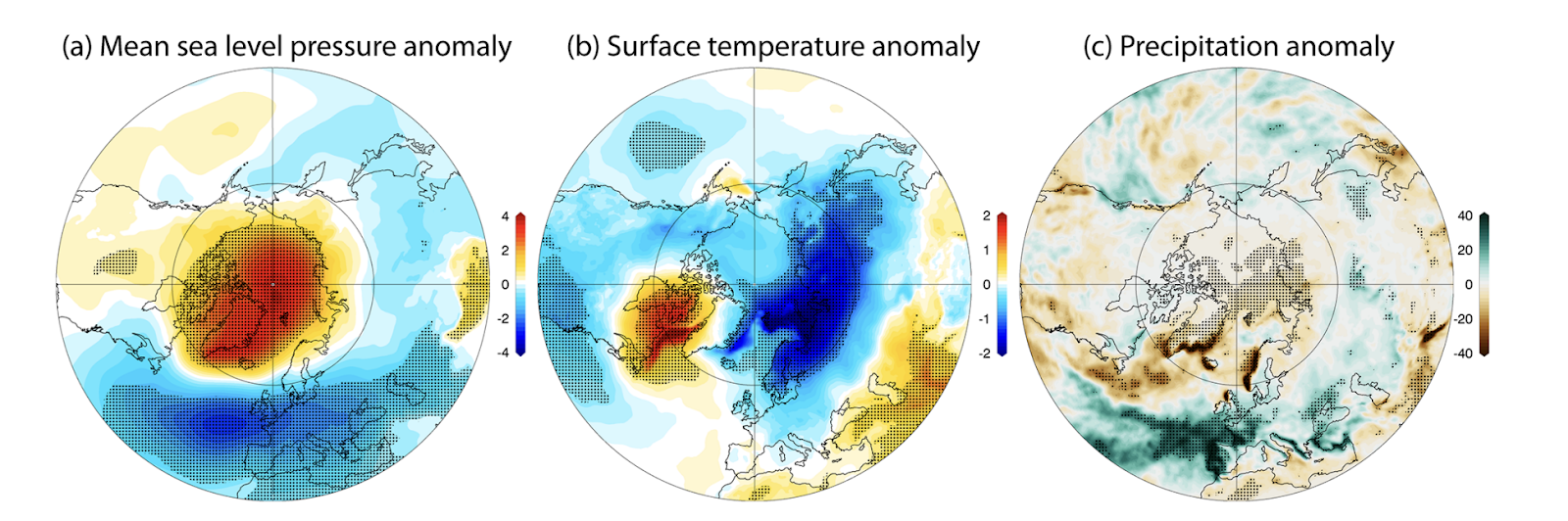
The interest in forecasting and modeling SSW events has spiked as studies continue to demonstrate the effects on surface weather. Despite the small effect size and the fact that not all SSW events result in noticeable weather changes, the phenomenon attracts attention, especially among the forecasting community.
Forecasting SSW Events - The Technical Challenges
What causes SSW events constitutes a growing area of research. The phenomenon demonstrates chaos theory in action, where small differences in initial conditions of the stratosphere can lead to a range of outcomes. Physics-based models like the European Centre for Medium-Range Weather Forecasts ensemble (ECMWF-ENS) often over-predict SSW events.
These physics-based models use observations about the current state of the stratosphere as inputs and evolve the current state forwards in time by integrating the equations that govern the physics of the atmosphere. Small errors in the observations can result in very different outcomes. Additionally, the atmospheric models have to use simplified versions of the governing equations in order to run in a reasonable time, and these simplifications (such as model vertical and horizontal resolution) ultimately result in model biases, such as overprediction of SSW events.
Obtaining a better current state of the atmosphere and better representation of the physics involves complex adjustments to both data assimilation systems and the atmospheric model. These changes require multiple experiments conducted by experts in the dynamics of the stratosphere and data assimilation. Thus it is a relatively slow process to correct the overprediction of SSW events. While a modeling center such as ECMWF may be aware of a bias, it will take a long time for it to be resolved.
Enter Salient, who calibrates physics-based inputs before integrating them into the Salient Blend forecasts. This results in forecast probabilities of SSW-triggered cold air outbreaks that reflect the actual likelihood of occurrence, and thus fewer false-alarms relative to uncalibrated forecasts. In addition to including physics-based models in the blended model, Salient also incorporates our own purely data-based AI model. Unlike physics-based modeling, which requires extensive expert knowledge and slow experimental integrations to improve accuracy, Salient’s proprietary AI model can be improved with a more agile methodology: by training on historical data, we can quickly adapt our models to the latest information, providing more accurate prediction of SSW impacts.
Why SSW Event Forecasting Matters
The significance of accurately predicting SSW events extends to industry. Energy traders, for instance, monitor these events closely due to their potential impact on weather patterns in Western Europe and North America, which influence energy prices. Salient's methodology not only corrects for the overconfidence often seen in physics-based forecasts, but produces the Salient AI model, which directly incorporates information from the stratosphere and other global data. This holistic approach allows for more refined temperature and precipitation forecasts, providing actionable insights for stakeholders. Salient's use of AI and machine learning to understand and forecast the impacts of SSW events represents a significant advancement in meteorological science, offering a more reliable foundation for weather prediction.
March 15, 2024
March 15, 2024
The Challenge & Importance of Forecasting Sudden Stratospheric Warming Events
Summary: Salient’s AI-powered methodology reduces bias in forecasting Sudden Stratospheric Warming (SSW) events, which are difficult to predict using physics-based models and important because they affect weather at the earth’s surface.

Introduction to Sudden Stratospheric Warming Events
The stratosphere begins 10 km above the surface; how can weather conditions so far from the surface impact weather on the ground? Research over the past 20 years has revealed that certain conditions can disrupt the stratospheric polar vortex – and this can indeed impact winter weather at Earth’s surface. Recently, research into the predictability of SSW events and their associated surface impacts has accelerated.
The polar vortex, a ring of strong winds circling the polar regions, can weaken and even split into two during Sudden Stratospheric Warming (SSW) events, pushing cold air south. Although challenging to predict, these occurrences can have significant impacts on global surface weather conditions. The statistically significant effects of SSW events (Figure 1) are most pronounced in northern Siberia, leading to colder temperatures, while Western Europe often experiences increased precipitation. Also, SSW events on average have a small but significant impact on the eastern United States, leading to colder-than-usual temperatures.
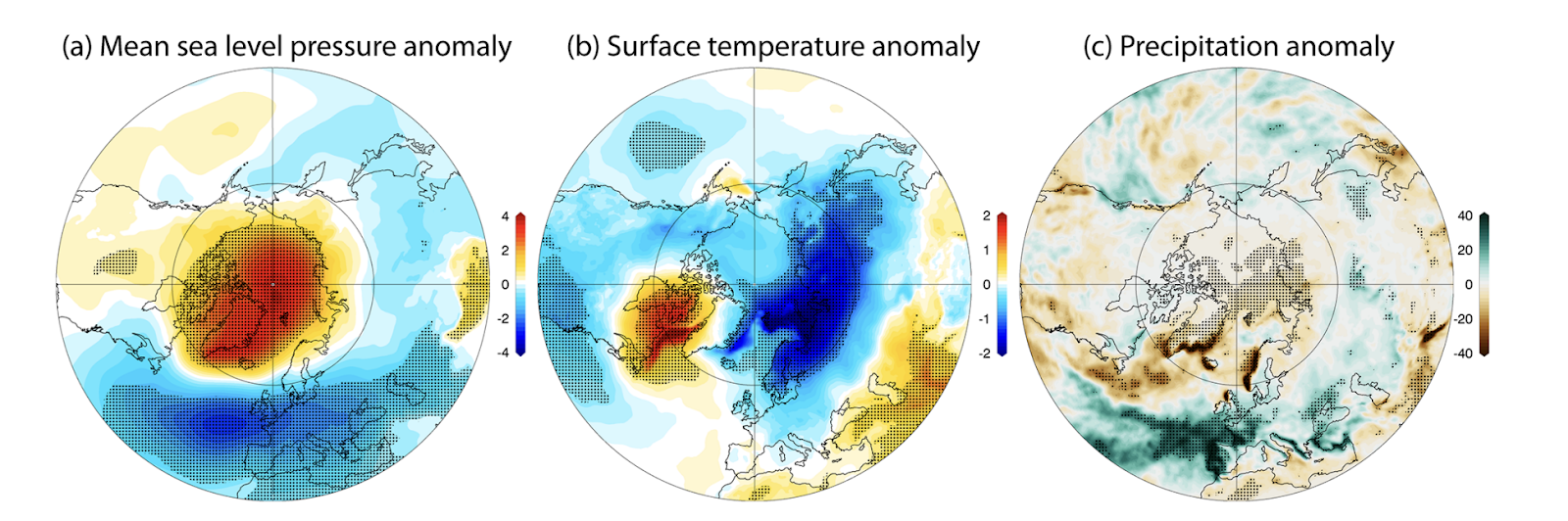
The interest in forecasting and modeling SSW events has spiked as studies continue to demonstrate the effects on surface weather. Despite the small effect size and the fact that not all SSW events result in noticeable weather changes, the phenomenon attracts attention, especially among the forecasting community.
Forecasting SSW Events - The Technical Challenges
What causes SSW events constitutes a growing area of research. The phenomenon demonstrates chaos theory in action, where small differences in initial conditions of the stratosphere can lead to a range of outcomes. Physics-based models like the European Centre for Medium-Range Weather Forecasts ensemble (ECMWF-ENS) often over-predict SSW events.
These physics-based models use observations about the current state of the stratosphere as inputs and evolve the current state forwards in time by integrating the equations that govern the physics of the atmosphere. Small errors in the observations can result in very different outcomes. Additionally, the atmospheric models have to use simplified versions of the governing equations in order to run in a reasonable time, and these simplifications (such as model vertical and horizontal resolution) ultimately result in model biases, such as overprediction of SSW events.
Obtaining a better current state of the atmosphere and better representation of the physics involves complex adjustments to both data assimilation systems and the atmospheric model. These changes require multiple experiments conducted by experts in the dynamics of the stratosphere and data assimilation. Thus it is a relatively slow process to correct the overprediction of SSW events. While a modeling center such as ECMWF may be aware of a bias, it will take a long time for it to be resolved.
Enter Salient, who calibrates physics-based inputs before integrating them into the Salient Blend forecasts. This results in forecast probabilities of SSW-triggered cold air outbreaks that reflect the actual likelihood of occurrence, and thus fewer false-alarms relative to uncalibrated forecasts. In addition to including physics-based models in the blended model, Salient also incorporates our own purely data-based AI model. Unlike physics-based modeling, which requires extensive expert knowledge and slow experimental integrations to improve accuracy, Salient’s proprietary AI model can be improved with a more agile methodology: by training on historical data, we can quickly adapt our models to the latest information, providing more accurate prediction of SSW impacts.
Why SSW Event Forecasting Matters
The significance of accurately predicting SSW events extends to industry. Energy traders, for instance, monitor these events closely due to their potential impact on weather patterns in Western Europe and North America, which influence energy prices. Salient's methodology not only corrects for the overconfidence often seen in physics-based forecasts, but produces the Salient AI model, which directly incorporates information from the stratosphere and other global data. This holistic approach allows for more refined temperature and precipitation forecasts, providing actionable insights for stakeholders. Salient's use of AI and machine learning to understand and forecast the impacts of SSW events represents a significant advancement in meteorological science, offering a more reliable foundation for weather prediction.
About Salient
Salient combines ocean and land-surface data with machine learning and climate expertise to deliver accurate and reliable subseasonal-to-seasonal weather forecasts and industry insights—two to 52 weeks in advance. Bringing together leading experts in physical oceanography, climatology and the global water cycle, machine learning, and AI, Salient helps enterprise clients improve resiliency, increase preparedness, and make better decisions in the face of a rapidly changing climate. Learn more at www.salientpredictions.com and follow on LinkedIn and X.