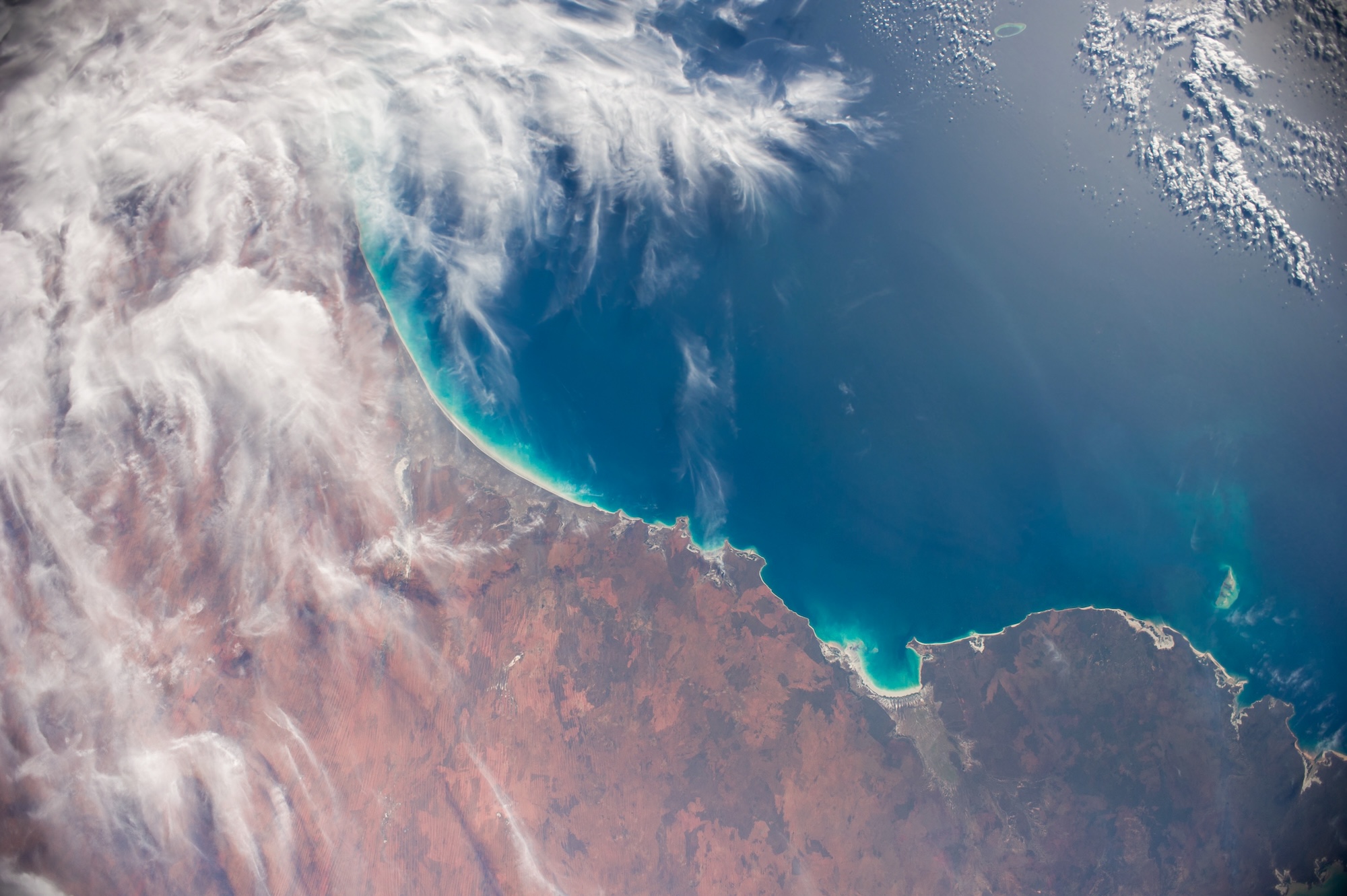
January 5, 2024
January 5, 2024
Unlocking Business Potential Through Advanced S2S Forecasting: Insights from NASEM’s 2023 Fall Meeting

At the annual fall meeting of the Board on Atmospheric Sciences and Climate (BASC) of the National Academy of Science, Engineering, and Medicine (NASEM), scientists discussed advancements in sub-seasonal to seasonal (S2S) forecasting since the 2016 recommendation to collectively achieve widespread use of S2S forecasts within the decade. Led by Brad Colman, atmospheric scientist and member of BASC, the meeting delved into the evolution of S2S predictive skill via data-driven methods (ex. machine-learning), and elucidated growing industry potential in S2S weather forecasting.
Just the Highlights
Considering where S2S weather forecasting has been and where it is going, Salient underlines three highlights from the conversation:
- Quantifying uncertainty is important.
- Thinking about Forecasts of Opportunity (FOO) is the way forward when it comes to the usability and usefulness of S2S forecasts.
- Trust and explainability go hand-in-hand for most end users of S2S forecasts.
The Deep Dive: Key Takeaways from NASEM’s Meeting
Advancements in AI: Andy Brown from the European Centre for Medium-Range Weather Forecasts (ECMWF) reflected on the collective progress since the 2016 BASC report. Most notably, the integration of artificial intelligence / machine learning (AI / ML) has been a game-changer for enhanced accuracy and reliability of S2S forecasts. Aneesh Subramanian (University of Colorado, Boulder) and others discussed how the emergence of these data-driven forecasting models (AI/ML) signifies not just improved accuracy but also forecasts that can be tailored to specific industry needs. AI's ability to draw trends from vast datasets of historical weather patterns has significantly increased the skill of S2S forecasts, allowing for more nuanced predictions that adapt to evolving atmospheric conditions. AI/ML-driven forecasts can also be customized to address unique challenges for different sectors. This progress is vital for industries and businesses that rely on weather forecasts for operational planning and risk mitigation.
The Power of Multi-Model Approaches
AI is not a perfect solution for S2S forecasting and demonstrates higher skill when blended with numerical weather prediction models (NWPs) that use mathematical models of the atmosphere and ocean to predict weather events. No single forecasting model is omniscient, and Ben Kirtman (University of Miami) and Kathy Pegion (University of Oklahoma) emphasized the effectiveness of multi-model ensemble approaches in reducing S2S forecast uncertainty. A multi-model approach that blends AI and NWP models with unique strengths and weaknesses often results in a more robust and reliable forecast (Salient’s forecasting takes the same approach). For businesses, this means forecasts they can trust, leading to more confident decision-making engines and, ultimately, increased profitability.

Understanding Sources of Predictability
Progress in S2S accuracy comes from understanding what produces reliable forecasts. For example, the “discard test” uses machine learning to remove low-confidence predictions from the dataset, producing a more reliable forecast. In addition, these deep neural networks involve millions of nonlinear relationships that contain powerful insights regarding what exactly makes a forecast prediction reliable. As Kirsten Mayer (National Center for Atmospheric Research) discussed, “explainable” or “interpretable” AI (XAI or IAI, respectively) can extract these complex relationships within the model data to identify sources of high and low predictability. Those “learned” indicators of predictability and other patterns can be validated against historical datasets in a process called reanalysis, which generates insights that drive the science of S2S forecasts forward and lay the groundwork for industry applications. These data-driven models evolve quickly, and weather-impacted businesses that employ advances in S2S forecasting anticipate resource availability and market shifts with greater precision, thereby creating or maintaining a competitive edge in a rapidly evolving landscape.
Collaboration is Key
Meeting presenters underscored the importance of interdisciplinary collaboration in accelerating S2S potential. Dr. Jason Furtado (University of Oklahoma), who also serves on Salient’s Science Advisory Board, put it this way: “There are experts in other areas that may not be in atmospheric science or the forecasting community [whose methodologies] we could impart into improving both our physics- and data-driven models.” For example, Salient’s forecasting model emerged from a collaboration between scientists at MIT and Woods Hole Oceanographic Institution.
Dr. Furtado, among others, also pointed to the invaluable role user and stakeholder input plays in generating trustful probabilistic S2S forecasts. Probabilistic forecasts can be hard to understand and even harder to extrapolate into a process. Salient collaborates with users across industries through co-production and improves forecasts to address specific use cases.
For example, forecasting anomalies alone is often insufficient for decision-making, so Salient translates probabilistic forecasts into risk alerts for when critical thresholds are exceeded. Collaboration with users tailors probabilistic forecasts to pain points and unveils new opportunities for commercial applications. Due to co-production, Salient model outputs translate readily across different industries and provide legible and useful forecasts to a range of stakeholders. Simply put: Successful S2S ventures collaborate continuously to create value.
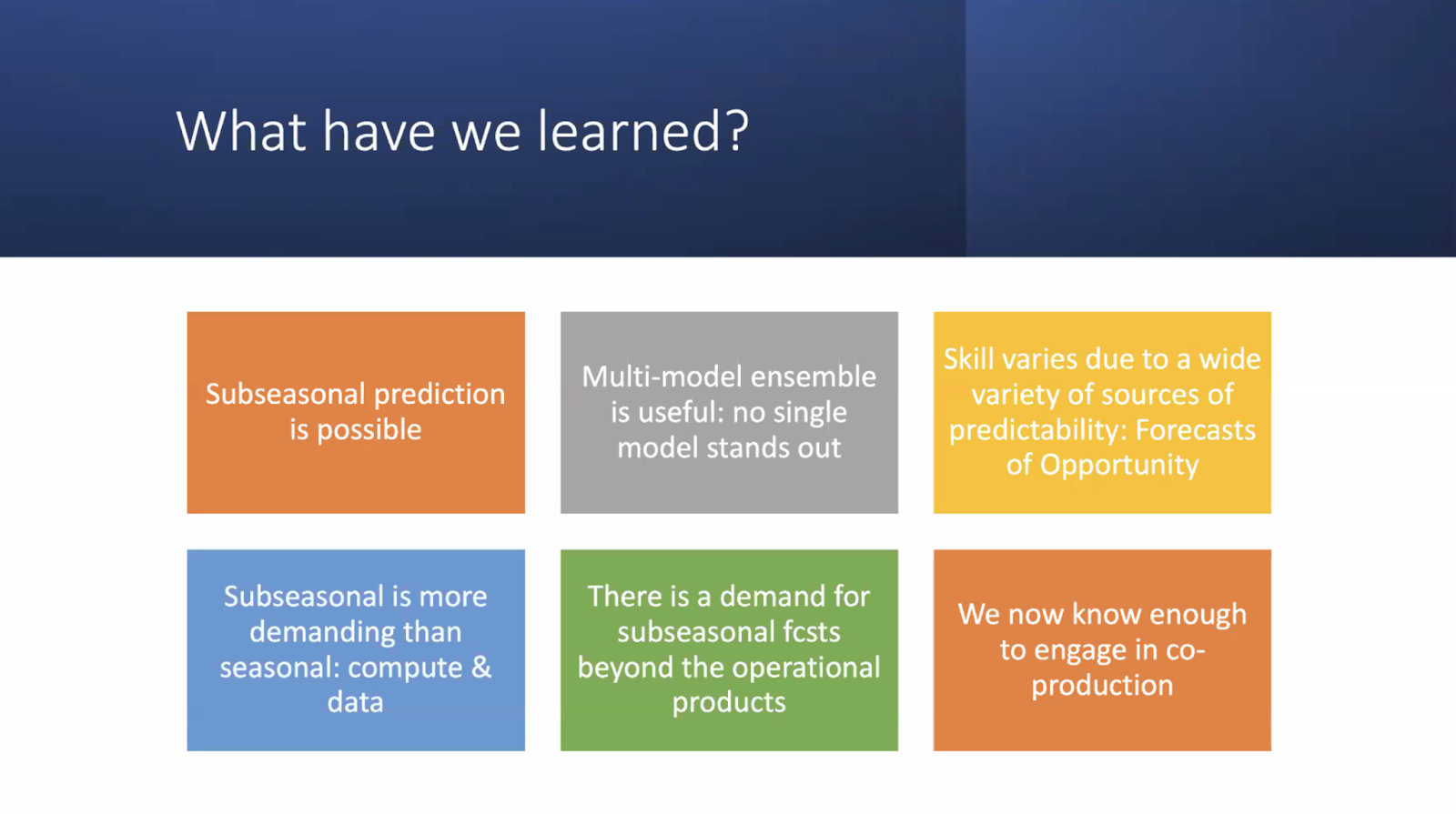
January 5, 2024
January 5, 2024
Unlocking Business Potential Through Advanced S2S Forecasting: Insights from NASEM’s 2023 Fall Meeting

At the annual fall meeting of the Board on Atmospheric Sciences and Climate (BASC) of the National Academy of Science, Engineering, and Medicine (NASEM), scientists discussed advancements in sub-seasonal to seasonal (S2S) forecasting since the 2016 recommendation to collectively achieve widespread use of S2S forecasts within the decade. Led by Brad Colman, atmospheric scientist and member of BASC, the meeting delved into the evolution of S2S predictive skill via data-driven methods (ex. machine-learning), and elucidated growing industry potential in S2S weather forecasting.
Just the Highlights
Considering where S2S weather forecasting has been and where it is going, Salient underlines three highlights from the conversation:
- Quantifying uncertainty is important.
- Thinking about Forecasts of Opportunity (FOO) is the way forward when it comes to the usability and usefulness of S2S forecasts.
- Trust and explainability go hand-in-hand for most end users of S2S forecasts.
The Deep Dive: Key Takeaways from NASEM’s Meeting
Advancements in AI: Andy Brown from the European Centre for Medium-Range Weather Forecasts (ECMWF) reflected on the collective progress since the 2016 BASC report. Most notably, the integration of artificial intelligence / machine learning (AI / ML) has been a game-changer for enhanced accuracy and reliability of S2S forecasts. Aneesh Subramanian (University of Colorado, Boulder) and others discussed how the emergence of these data-driven forecasting models (AI/ML) signifies not just improved accuracy but also forecasts that can be tailored to specific industry needs. AI's ability to draw trends from vast datasets of historical weather patterns has significantly increased the skill of S2S forecasts, allowing for more nuanced predictions that adapt to evolving atmospheric conditions. AI/ML-driven forecasts can also be customized to address unique challenges for different sectors. This progress is vital for industries and businesses that rely on weather forecasts for operational planning and risk mitigation.
The Power of Multi-Model Approaches
AI is not a perfect solution for S2S forecasting and demonstrates higher skill when blended with numerical weather prediction models (NWPs) that use mathematical models of the atmosphere and ocean to predict weather events. No single forecasting model is omniscient, and Ben Kirtman (University of Miami) and Kathy Pegion (University of Oklahoma) emphasized the effectiveness of multi-model ensemble approaches in reducing S2S forecast uncertainty. A multi-model approach that blends AI and NWP models with unique strengths and weaknesses often results in a more robust and reliable forecast (Salient’s forecasting takes the same approach). For businesses, this means forecasts they can trust, leading to more confident decision-making engines and, ultimately, increased profitability.

Understanding Sources of Predictability
Progress in S2S accuracy comes from understanding what produces reliable forecasts. For example, the “discard test” uses machine learning to remove low-confidence predictions from the dataset, producing a more reliable forecast. In addition, these deep neural networks involve millions of nonlinear relationships that contain powerful insights regarding what exactly makes a forecast prediction reliable. As Kirsten Mayer (National Center for Atmospheric Research) discussed, “explainable” or “interpretable” AI (XAI or IAI, respectively) can extract these complex relationships within the model data to identify sources of high and low predictability. Those “learned” indicators of predictability and other patterns can be validated against historical datasets in a process called reanalysis, which generates insights that drive the science of S2S forecasts forward and lay the groundwork for industry applications. These data-driven models evolve quickly, and weather-impacted businesses that employ advances in S2S forecasting anticipate resource availability and market shifts with greater precision, thereby creating or maintaining a competitive edge in a rapidly evolving landscape.
Collaboration is Key
Meeting presenters underscored the importance of interdisciplinary collaboration in accelerating S2S potential. Dr. Jason Furtado (University of Oklahoma), who also serves on Salient’s Science Advisory Board, put it this way: “There are experts in other areas that may not be in atmospheric science or the forecasting community [whose methodologies] we could impart into improving both our physics- and data-driven models.” For example, Salient’s forecasting model emerged from a collaboration between scientists at MIT and Woods Hole Oceanographic Institution.
Dr. Furtado, among others, also pointed to the invaluable role user and stakeholder input plays in generating trustful probabilistic S2S forecasts. Probabilistic forecasts can be hard to understand and even harder to extrapolate into a process. Salient collaborates with users across industries through co-production and improves forecasts to address specific use cases.
For example, forecasting anomalies alone is often insufficient for decision-making, so Salient translates probabilistic forecasts into risk alerts for when critical thresholds are exceeded. Collaboration with users tailors probabilistic forecasts to pain points and unveils new opportunities for commercial applications. Due to co-production, Salient model outputs translate readily across different industries and provide legible and useful forecasts to a range of stakeholders. Simply put: Successful S2S ventures collaborate continuously to create value.
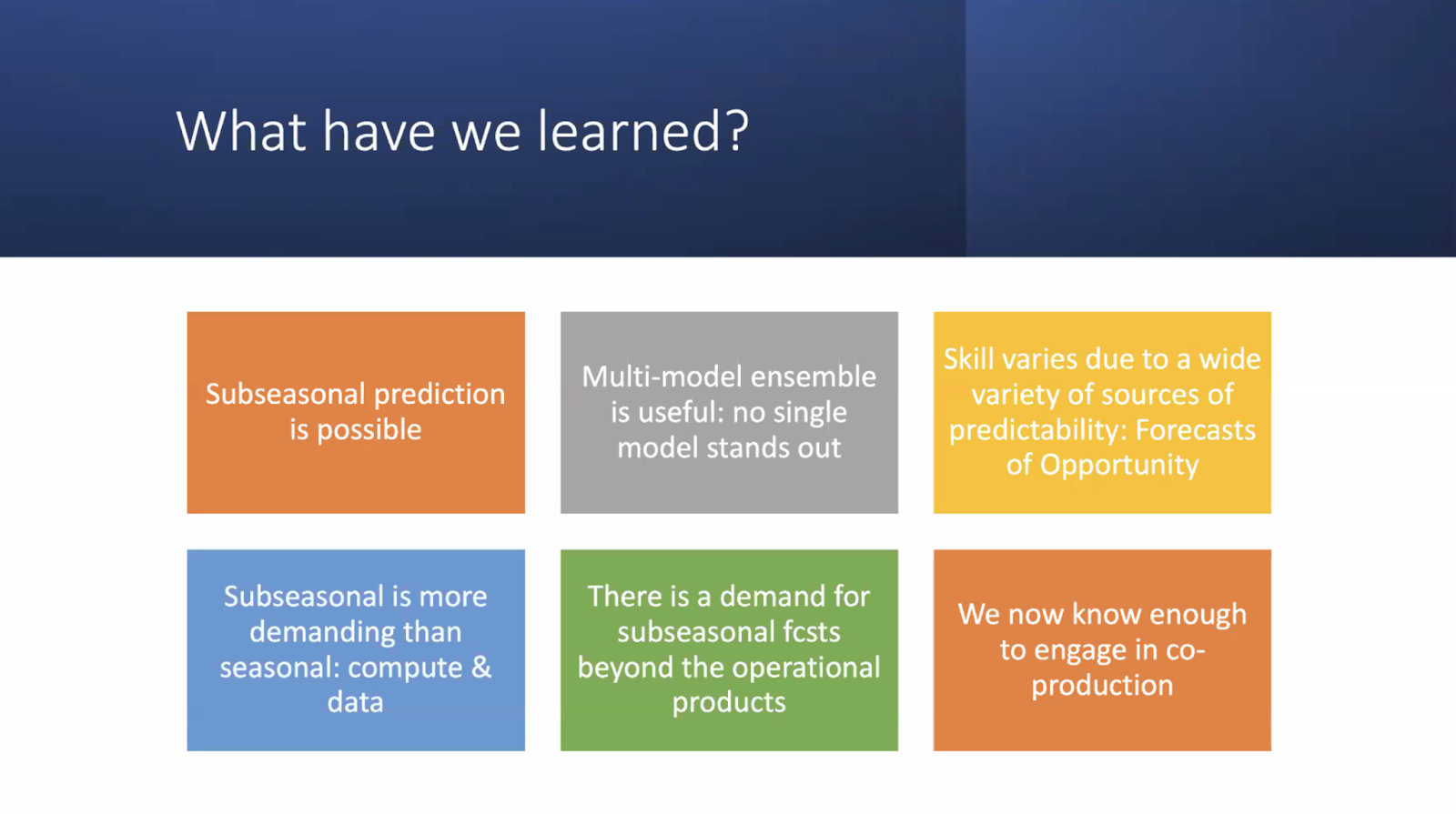
About Salient
Salient combines ocean and land-surface data with machine learning and climate expertise to deliver accurate and reliable subseasonal-to-seasonal weather forecasts and industry insights—two to 52 weeks in advance. Bringing together leading experts in physical oceanography, climatology and the global water cycle, machine learning, and AI, Salient helps enterprise clients improve resiliency, increase preparedness, and make better decisions in the face of a rapidly changing climate. Learn more at www.salientpredictions.com and follow on LinkedIn and X.